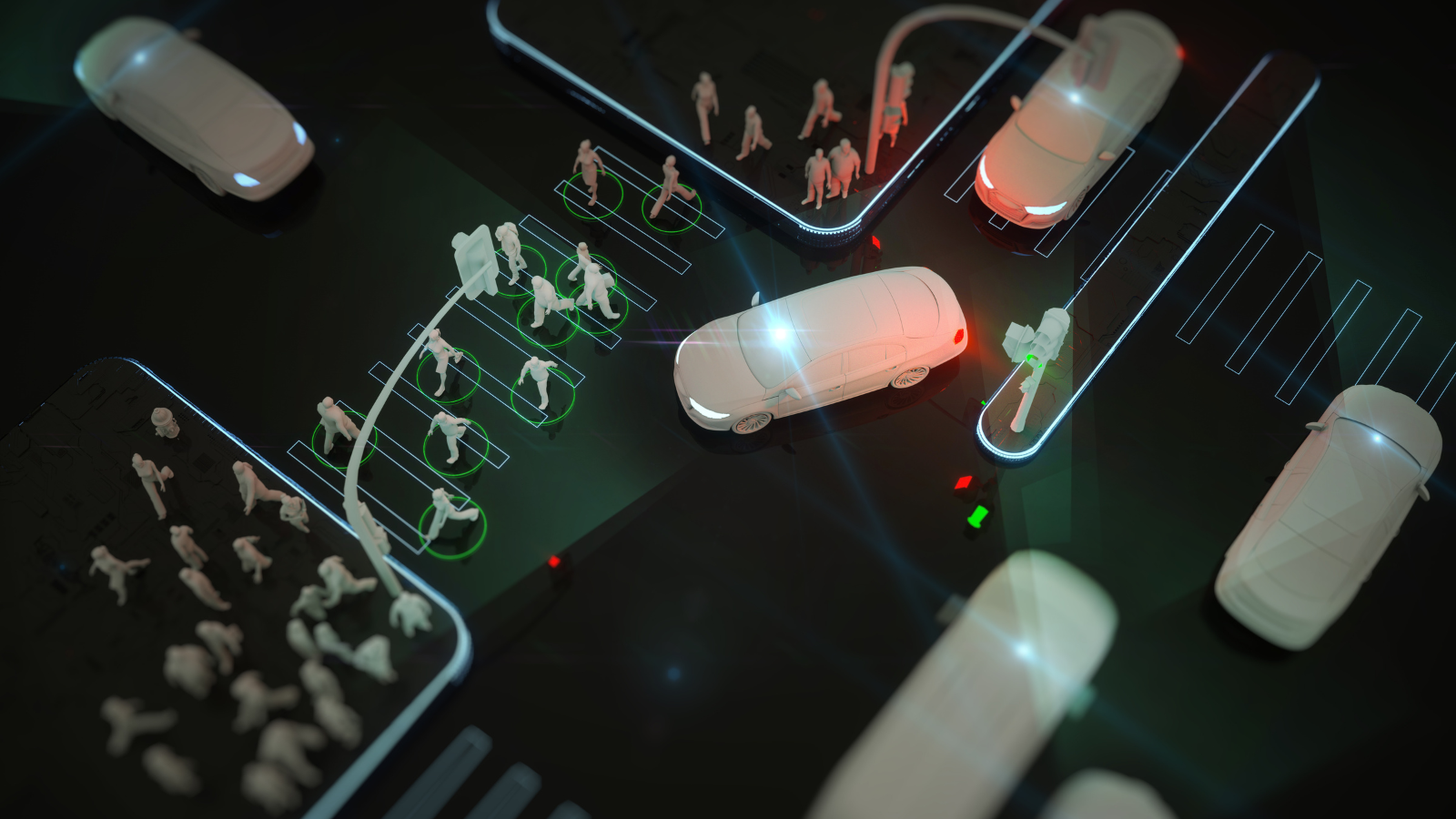
A framework based on Deep Learning for feature extraction
This paper by Wen et al., presents a a deep learning framework for road marking extraction, completion and classification from 3D mobile laser scanning point clouds.
This research presented by Wen et al., A showcases a deep learning-based framework for road marker extraction, classification, and completion from three-dimensional (3D) mobile laser scanning (MLS) point clouds.
Road markers are one of the most essential components for guiding autonomous vehicles and play a crucial role in road traffic safety (AVs).
The method used in this study is less sensitive to data quality than existing road marker extraction techniques, which are mostly reliant on intensity thresholds. The step of road marking completion was added to further optimize the results.
To overcome concerns with intensity variations, low contrast, and other issues, a modified U-net model was utilized to segment road marking pixels during the extraction stage.
In order to classify the various types of road markings with significant differences, a hierarchical classification method integrating multi-scale clustering with Convolutional Neural Networks (CNN) was created.
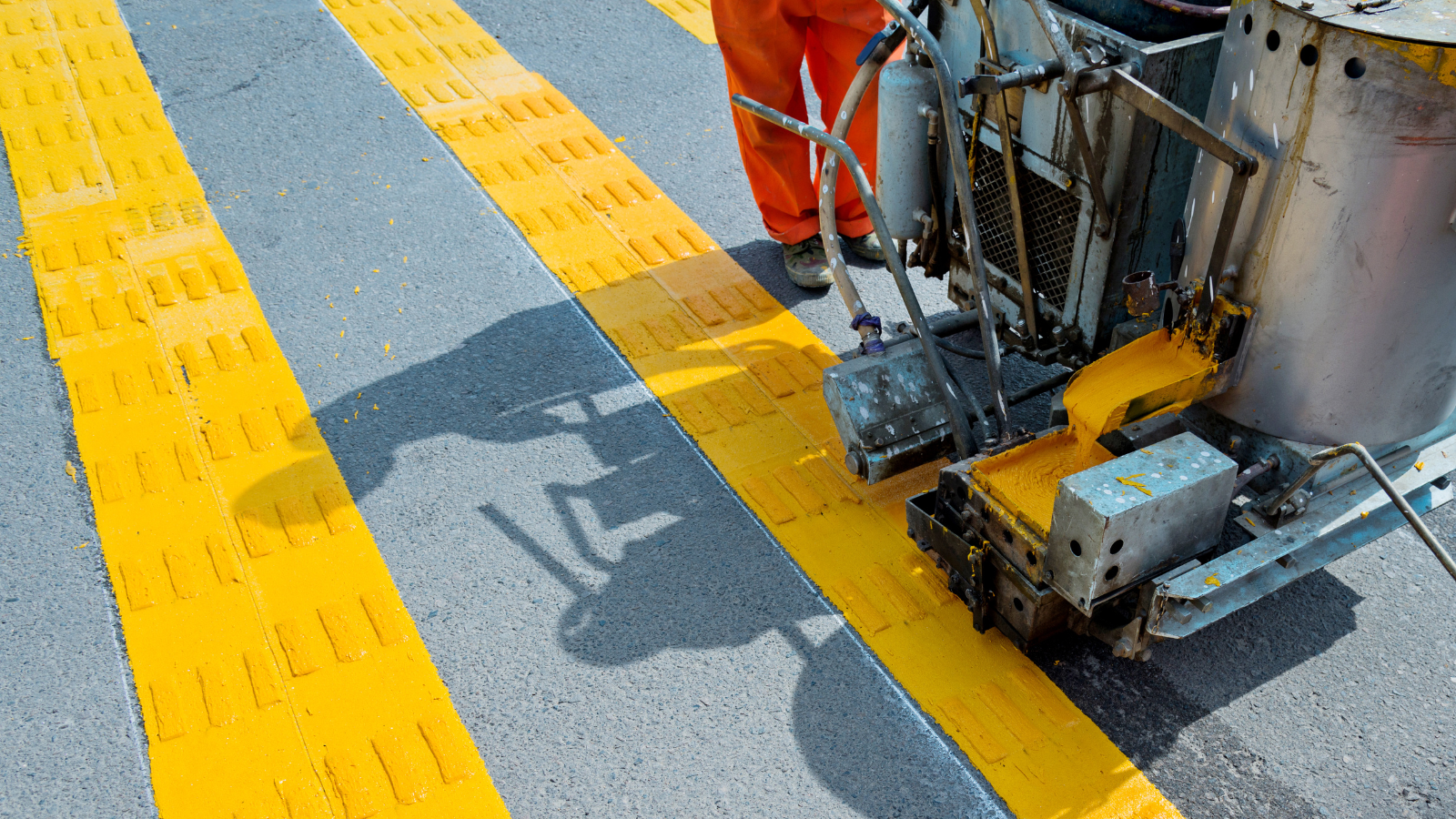
At the completion stage, a method based on a Generative Adversarial Network (GAN) was developed to complete small-size road markings first, then followed by completing broken lane lines and adding missing markings using a context-based method.
Additionally, in order to train the deep network model and assess the methodology, a point cloud road marking dataset was built.
The experimental findings, which were acquired using point clouds from various scenes, demonstrated that the method proposed is very promising for the road marking extraction, classification, and completion.
Abstract
Road markings play a critical role in road traffic safety and are one of the most important elements for guiding autonomous vehicles (AVs).
High-Definition (HD) maps with accurate road marking information are very useful for many applications ranging from road maintenance, improving navigation, and prediction of upcoming road situations within AVs.
This paper presents a deep learning-based framework for road marking extraction, classification and completion from three-dimensional (3D) mobile laser scanning (MLS) point clouds.
Compared with existing road marking extraction methods, which are mostly based on intensity thresholds, our method is less sensitive to data quality.
We added the step of road marking completion to further optimize the results.
At the extraction stage, a modified U-net model was used to segment road marking pixels to overcome the intensity variation, low contrast and other issues.
At the classification stage, a hierarchical classification method by integrating multi-scale clustering with Convolutional Neural Networks (CNN) was developed to classify different types of road markings with considerable differences.
At the completion stage, a method based on a Generative Adversarial Network (GAN) was developed to complete small-size road markings first, then followed by completing broken lane lines and adding missing markings using a context-based method. In addition, we built a point cloud road marking dataset to train the deep network model and evaluate our method.
The dataset contains urban road and highway MLS data and underground parking lot data acquired by our own assembled backpacked laser scanning system.
Our experimental results obtained using the point clouds of different scenes demonstrated that our method is very promising for road marking extraction, classification and completion.

LiDAR INSIGHTER Newsletter
Join the newsletter to receive the latest updates in your inbox.